Join As Students, Leave As Professionals.
Develearn is the best institute in Mumbai, a perfect place to upgrade your skills and get yourself to the next level. Enroll now, grow with us and get hired.
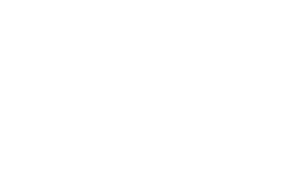
The Importance of Vector Databases for LLM Empowerment
This blog explores the critical role that vector databases play in LLM empowerment, shedding light on their capabilities and the advantages they bring to the legal field. From improving legal research to enhancing case management, vector databases are revolutionizing the way legal professionals operate.
Vector
Data Base
LLM
Develearn
3 minutes
September 12, 2023
Introduction
Vector Databases are a rising star in the field of contemporary data management. Due to their distinctive capabilities and uses, these cutting-edge data storage systems have drawn the interest of both researchers and professionals. But what precisely are Vector Databases, and why are LLMs so dependent on them? In this blog article, we’ll explain what vector databases are and how they play a crucial part in boosting LLMs’ capabilities.
Whats is Vector Databases?
Vector databases are fundamentally made for the purpose of effectively managing and storing vectorized data. In this context, a vector denotes a collection of numerical values that accurately encapsulate the characteristics and qualities of a data item. Vector Databases are able to succeed in situations where data analysis, similarity search, and intricate mathematical computations are crucial because to their ground-breaking method of data storing.
Important information for LLMs:
Large Language Models, like the GPT-3.5 architecture, work on enormous datasets and carry out intricate tasks involving language. Here’s why vector databases are necessary to improve the capabilities of LLMs:
1. A quick similarity search is:
Within huge databases, LLMs frequently have to look for related text snippets or documents. Due of the enormous dimensionality of linguistic data, conventional databases have trouble handling this task. On the other hand, vectorized representations are used by vector databases to quantify similarities precisely and effectively. This capacity enables LLMs to quickly obtain pertinent information and conduct contextual searches.
2. Improved Contextual Knowledge:
In order to grasp a language, context is essential. In capturing complex relationships and context inside data, vector databases shine. This has implications for LLMs since it allows the model to understand subtleties, adapt to changes in context, and give more precise answers. It completely alters how human-like language comprehension is delivered.
3. Exploring Real-Time Data:
LLMs need rapid access to vast knowledge bases. By enhancing query performance and shortening retrieval times, vector databases allow for quick data exploration. When creating content, responding to questions, or making suggestions, LLMs may seamlessly connect with consumers because to this real-time information access.
4. Training Data Effectiveness:
Huge volumes of data are analyzed throughout the LLM training phase in order to create a solid language model. By enabling effective training data storage, retrieval, and manipulation, vector databases improve this procedure. As a consequence, model training takes place more quickly, and computing resources are used more effectively.
5. Applications for Multimodality:
Vector databases can hold vectorized representations of pictures, audio, and other modalities in addition to text data. This creates the foundation for multimodal applications where LLMs may easily combine language comprehension with other types of data processing, resulting in more engaging user interfaces.
Conclusion
In conclusion, the capabilities of Large Language Models are strongly impacted by the revolutionary development in data storage that is represented by Vector Databases. The complexity of language interpretation and context precisely coincides with their capacity to store and retrieve vectorized material quickly. The addition of Vector Databases to LLM operations provides a level of efficiency, precision, and depth as LLMs continue to change how we interact with technology. These databases provide as evidence of the growing interoperability of AI technology, indicating a potential future of improved language comprehension and communication.