Join As Students, Leave As Professionals.
Develearn is the best institute in Mumbai, a perfect place to upgrade your skills and get yourself to the next level. Enroll now, grow with us and get hired.
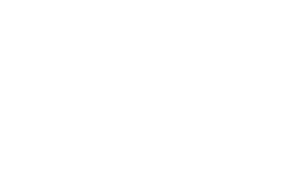
Top 6 NLP Techniques Every Data Scientist Should Know
Best 6 Different Natural language processing (NLP) Techniques-List of the basic NLP techniques python that every data scientist or machine learning engineer should know.
NLP Techniques
Data Scientist
Education
DeveLearn
3 minutes
November 1, 2023
Top 6 NLP Techniques Every Data Scientist Should Know
Natural language processing (NLP) has transformed data analytics across various industries, becoming an integral part of technological advancements. Examples of NLP in action are abundant and can be observed everywhere. However, the success or failure of your business in today's competitive market hinges on how effectively you leverage natural language processing.
This article explores practical ways to enhance your NLP practices, providing insights to help you navigate the dynamic landscape of modern business. Whether you prefer to read through the entire article at your own pace or directly dive into specific natural language processing techniques, the optimization of these practices is crucial for streamlining your business operations.
What is Natural Language Processing (NLP)?
Natural Language Processing (NLP) in data science refers to the automated manipulation of natural languages, such as speech and text, using software to enable computers to observe, analyze, understand, and extract meaningful insights from human-spoken languages. Essentially, NLP is a specialized branch of data science dedicated to teaching computers to process and interpret text-based conversations in a manner akin to human comprehension.
This field plays a vital role in bridging the gap between Data Science and human languages, presenting unique challenges during development. Unlike structured and unambiguous programming languages like Java or Python, human-spoken languages are inherently ambiguous and subject to change based on regional or social variations. Consequently, training computers to comprehend natural languages proves to be a demanding task.
NLP applications are crucial components of data science, and a comprehensive Data Science Certification program should ideally include a live project on NLP.
Why is Natural Language Processing Important?
Natural Language Processing (NLP) holds significant importance for several reasons. Picture your business software communicating in a foreign language you're not fluent in – that's where NLP steps in as your translator. It takes your human input, rearranges it, and articulates what you've conveyed in a manner that your software can comprehend.
Why should you be concerned? Communication is fundamental, and NLP software plays a pivotal role in enhancing how businesses function, ultimately influencing customer experiences positively. The ability of NLP to bridge the gap between human language and machine understanding is transformative, ensuring smoother interactions and more effective utilization of technology in various industries.
Now, let's dive deeper into understanding the six most used Natural Language Processing (NLP) Techniques in Data Science and how you can leverage them.
Top 6 Natural Language Processing (NLP) Techniques in Data Science
A branch of artificial intelligence called “Natural Language Processing” (NLP) focuses on how computers and human language interact. Textual data is widely available nowadays, thus NLP has become a crucial ability for data scientists. Every data scientist should be knowledgeable with the following six fundamental NLP techniques:
1. Tokenization
Tokenization is the process of dividing text into tokens, which are single words, phrases, or symbols. Because it serves as the foundation for several later tasks including text analysis, sentiment analysis, and machine translation, this method is crucial to NLP. Tokenization may be as basic as dividing text into spaces or it can be more complicated, taking special characters and punctuation into account.
2. Stop word Removal:
Common words like “the,” “and,” “is,” and “in” that often appear in big quantities but convey little useful information are known as “stopwords” in NLP. A significant preprocessing step to minimize noise in text data and make it more manageable for analysis is the removal of stopwords. Stopword lists for many languages are specified by libraries like NLTK (Natural Language Toolkit).
3. Lemmatization and Stemming:
Lemmatization and stemming are methods for reducing words to their root or fundamental form. This procedure assists in combining similar terms and lowering text data dimensionality. While lemmatization uses more complex linguistic procedures to reach the base form (e.g., “better” becomes “good”), stemming includes eliminating prefixes or suffixes to achieve the root form (for example, “running” becomes “run”).
4. Named Entity Recognition (NER)
NER is a method for locating and categorizing named entities (such as names of individuals, groups, places, dates, and more) in text. Sentiment analysis, document classification, and information extraction all depend on NER. Entities in many languages and topics may be identified using advanced NER models.
5. Word Embeddings
Techniques used to represent words in a vector space include Word2Vec, GloVe, and FastText. By capturing the semantic connections between words in these representations, text context and meaning may be understood by machines. A variety of NLP activities, such as sentiment analysis, document categorization, and machine translation, require word embeddings.
6.Sentiment Analysis:
Finding the sentiment or emotional tone represented in a text is the goal of sentiment analysis, commonly referred to as opinion mining. It divides text into positive, negative, and neutral categories. Sentiment analysis has uses in brand reputation management, social media monitoring, and analysis of consumer reviews.
Conclusion
Just the tip of the iceberg in the field of natural language processing are these six NLP approaches. It’s important to keep up with the most recent developments in the area as data scientists continue to investigate and create new NLP models and techniques. Mastering these fundamental methods is a great place to start for anybody wishing to deal with textual data and use the power of language in data science initiatives. NLP is a vibrant and developing field of study.